Implementing new technologies, including artificial intelligence, may seem like a quick and easy way to improve company performance. However, reality is often more complex, and many myths surrounding this process can lead to significant mistakes. Is open source really a haven for hackers? Is newly implemented software always ready to operate immediately? And finally – does AI implementation necessarily require extensive time? We already know the answers to these questions and are ready to share them with you.
Myth 1: Open source is a gateway for cybercriminals
There is a common belief that open-source software is easy to hack because the source code is accessible to everyone. Reality, however, disproves this myth. Penetration tests show that hacking an application with non-public source code is not necessarily harder, although it may take a little more time.
Moreover, if a company uses open-source software as a foundation for building its own solutions, it must ensure its code is updated to stay compatible with updates made to the original open-source software.
Myth 2: New solutions are ready for immediate deployment
On the other end of the spectrum, some organizations approach new technology implementation without a strategic plan, viewing it as a cure-all for the company’s issues. This approach presents various risks. How can they be avoided, and what mistakes should be prevented?
IT system security should be considered on two levels:
- Caution and vigilance to prevent unauthorized access.
- System stability, which can be ensured by implementing test procedures, including:
- automated tests,
- manual tests,
- simulation tests,
- load tests.
For example, in manufacturing companies implementing new software, testing is divided into stages. Automated tests come first, allowing programmers to test the software. After unit testing, manual and user testing follows.
Finally, the software is tested on the company server. Such simulations use fictitious data, so potential errors do not impact production.
Different user groups, including operators, can participate in these tests, both training on the simulated system and testing it.
After these stages, scenarios are created to test software using actual production data. Load tests in manufacturing help predict system stability in a real environment.
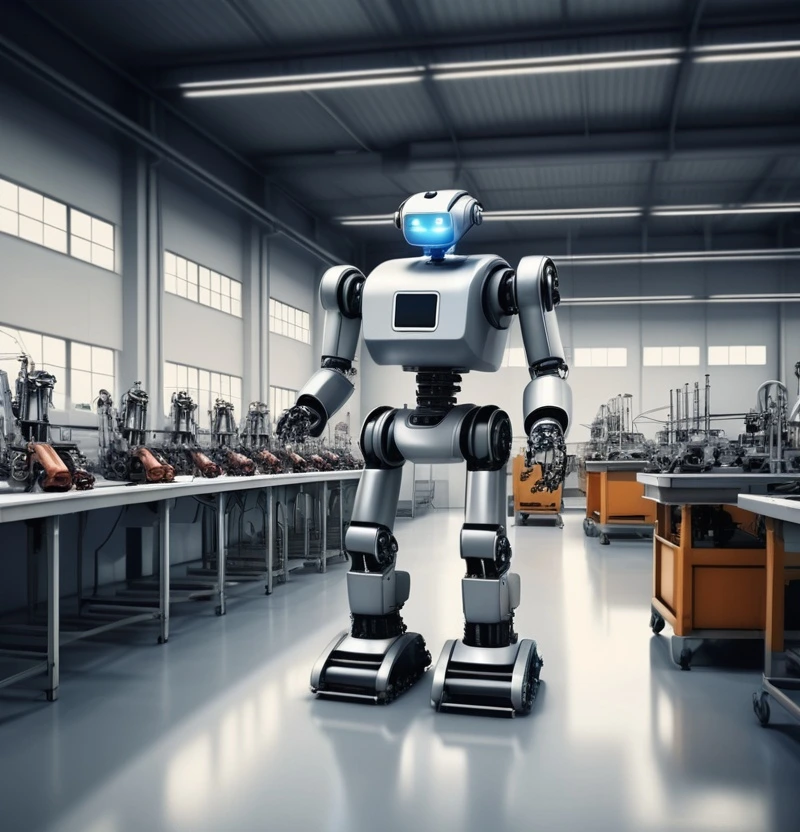
Myth 3: AI implementation is always long and complicated
A major issue in adopting new technologies in the B2B model is the prolonged and overly cautious decision-making process. AI is no exception here.
Many companies approach testing new technology in the wrong way, assuming they need to build a complete concept that solves all problems and anticipates every scenario. However, it’s far more effective to start with a pilot project to test a hypothesis on a small scale, giving insight into how well the software can solve specific issues.
During testing, companies often discover other areas where the technology could help and address unforeseen problems. This agile approach differs from attempting to plan software end-to-end before implementation. Hence, quicker decisions for a smaller pilot project, covering about 5–10% of the full scale, are beneficial, as they allow safe and budget-friendly testing of the solution’s viability.
In the case of AI implementation, many tools can conduct analytics by simply connecting the right data sources.
However, the foundation of AI solutions should be high-quality, well-prepared data. Each company must invest time in designing a database structure that serves as a reliable AI model, resulting in a robust and dependable system.
Preparing for potential risks in AI implementation
Implementing AI-driven systems has become a trend, attractive for drawing clients, though sometimes that’s all it is. It’s essential first to evaluate if the selected technology fits the problem and if the investment is worthwhile.
From problem to solution
We live in times when certain problems can be solved in various ways. What’s crucial is whether we choose a solution that addresses the problem or tailor the problem to fit a solution. Sometimes companies implement AI only to then consider what issues it could solve.
The foundation: databases
If an AI solution is genuinely necessary, the key component is always the database. If data lacks resolution or structure to provide context, the implementation will be ineffective.
This is not to discourage companies from AI-based solutions but to encourage thoughtful consideration, ensuring the chosen technology effectively addresses a particular problem, is scalable, cost-effective, and relevant. (If, for example, evidence shows a solution will soon become obsolete, it may not be worth investing in.)
Want to Implement New Solutions Successfully? Prepare a Strategy:
- Update your company’s testing procedures!
- Conduct data quality analysis before AI implementation!
- Launch a pilot project for the new technology!
Let these three steps help you debunk the three technology myths outlined above!
If you have questions about industrial digitization, explore explitia’s technology support.